کاربرد تکنیک های هوش مصنوعی برای تشخیص بیماری کبد چرب غیر الکلی: مروری سیستماتیک 2005-2023
بیماری کبد چرب غیر الکلی (NAFLD) یک بیماری شایع کبدی با شیوع سریع و در حال رشد در سراسر جهان است. برای پیش آگهی و تصمیمات درمانی، تشخیص مراحل پاتولوژیک NAFLD از جمله استئاتوز، استئاتوهپاتیت و فیبروز کبد مهم بوده و به طور قطعی در بیوپسی تهاجمی تشخیص داده میشوند. تصویربرداری سونوگرافی غیر تهاجمی (US) شامل تکنیک الاستوگرافی ایالات متحده و پارامترهای بالینی می تواند برای تشخیص و درجهبندی NAFLD و عوارض آن استفاده شود.
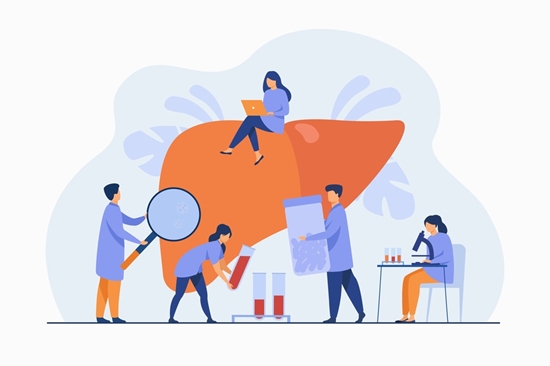
هوش مصنوعی (AI) به طور فزایندهای برای توسعه مدلهای تشخیصی NAFLD بر اساس دادههای بالینی، نشانگرهای زیستی یا تصویربرداری مورد استفاده قرار می گیرد. این مقاله جدید که در ScienceDirect منتشر شده است، به بررسی این موضوع میپردازد که چگونه هوش مصنوعی (AI) ممکن است جایگزین ایمنتر و در دسترستری برای تشخیص این بیماری ارائه دهد. همچنین به پتانسیل تکنیکهای هوش مصنوعی برای تشخیص NAFLD میپردازد.
در این مرور سیستماتیک محققان مجموعه ای از مطالعات موجود (از سال 2005 تا 2023) را در مورد استفاده از هوش مصنوعی برای تشخیص NAFLD تجزیه و تحلیل کردند و با تمرکز بر روی مدلهای هوش مصنوعی، از انواع مختلف دادهها مانند تصویربرداری اولتراسوند، آزمایش خون و سابقه بیمار برای شناسایی NAFLD و حتی پیشبینی شدت آن استفاده میکنند. این مطالعه نشان میدهد که هوش مصنوعی میتواند نقش اساسی و تغییردهنده برای تشخیص NAFLD تبدیل شود، زیرا میتوانند روشی غیرتهاجمی و بالقوه دقیقتر برای تشخیص NAFLD ارائه دهند که منجر به مراقبت بهتر از بیمار و مدیریت این بیماری رایجتر میشود.
Abstract
Background and objectives
Non-alcoholic fatty liver disease (NAFLD) is a common liver disease with a rapidly growing incidence worldwide. For prognostication and therapeutic decisions, it is important to distinguish the pathological stages of NAFLD: steatosis, steatohepatitis, and liver fibrosis, which are definitively diagnosed on invasive biopsy. Non-invasive ultrasound (US) imaging, including US elastography technique, and clinical parameters can be used to diagnose and grade NAFLD and its complications. Artificial intelligence (AI) is increasingly being harnessed for developing NAFLD diagnostic models based on clinical, biomarker, or imaging data. In this work, we systemically reviewed the literature for AI-enabled NAFLD diagnostic models based on US (including elastography) and clinical (including serological) data.
Methods
We performed a comprehensive search on Google Scholar, Scopus, and PubMed search engines for articles published between January 2005 and June 2023 related to AI models for NAFLD diagnosis based on US and/or clinical parameters using the following search terms: “non-alcoholic fatty liver disease”, “non-alcoholic steatohepatitis”, “deep learning”, “machine learning”, “artificial intelligence”, “ultrasound imaging”, “sonography”, “clinical information”.
Results
We reviewed 64 published models that used either US (including elastography) or clinical data input to detect the presence of NAFLD, non-alcoholic steatohepatitis, and/or fibrosis, and in some cases, the severity of steatosis, inflammation, and/or fibrosis as well. The performances of the published models were summarized, and stratified by data input and algorithms used, which could be broadly divided into machine and deep learning approaches.
Conclusion
AI models based on US imaging and clinical data can reliably detect NAFLD and its complications, thereby reducing diagnostic costs and the need for invasive liver biopsy. The models offer advantages of efficiency, accuracy, and accessibility, and serve as virtual assistants for specialists to accelerate disease diagnosis and reduce treatment costs for patients and healthcare systems.
ارسال به دوستان