چالشهای توسعه و اعتبارسنجی مدلهای ماشین لرنینگ برای پیشبینی خطر مرگ و میر کاشت دریچه آئورت ترانس کاتتر
این مقاله مشکلات موجود در ایجاد و آزمایش برنامههای رایانهای را مورد بحث قرار میدهد که از دادههای بیمار برای پیشبینی خطر مرگ پس از یک عمل قلبی به نام کاشت دریچه آئورت ترانس کاتتر (TAVI) استفاده میکنند.
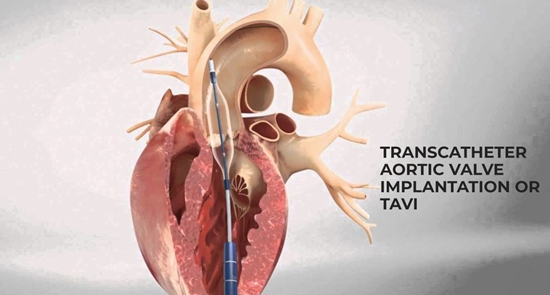
در حالی که این برنامه ها نویدبخش بهبود مراقبت از بیمار هستند، چالش هایی برای اطمینان از دقیق و قابل اعتماد بودن آنها وجود دارد. این مطالعه این چالش ها را به گونه ای مورد بررسی قرار می دهد که برای عموم مردم قابل درک باشد.
Challenges in developing and validating machine learning models for transcatheter aortic valve implantation mortality risk prediction
Model selection
In this study, the authors evaluated several ML models and utilized random forest models as the best-performing model, yet they did not report the performance metrics such as area under the curve (AUC) of alternative models.1 The comparison between different ML models (such as logistic regression, support vector machines, decision trees, gradient boosting machines, and neural networks) can help identify potential biases and limitations in the developed predictive score.2 Furthermore, it allows for the selection of a model that best suits the complexity of the data and would allow readers to better assess the robustness and generalizability of the selected model.
ارسال به دوستان